Content Analysis
- Antonio Jimenez
- Dec 1, 2023
- 11 min read
What is Content Analysis?
Content analysis is a methodical and detailed examination of materials created by people, such as documents, photographs, or even street signs, to identify patterns, themes, and meanings. These materials, referred to as social artifacts, are not typically intended for research but can reveal insights into various cultural and social aspects. The process involves coding this content into data to address specific research questions.
For instance, content analysis can be used to assess the tone of language in advertisements, detect stereotypes in textbooks across decades, analyze the influence of cultural narratives in literature, or study the communication styles of political candidates. It's a versatile tool that can apply to diverse fields like sociology, criminology, psychology, and more.
An example of content analysis is Amy Binder's study of how “dangerous” music was portrayed in news articles between 1985 and 1990. She found that heavy metal and rap music were framed differently, with heavy metal being seen as morally corruptive (presumably to white listeners) and rap as a societal danger (implying harm from black listeners).
Content analysis also allows for the study of long-term societal trends. For example, McBroom's study of the portrayal of women in the clergy in the Christian Century magazine revealed a shift from initial positive references to predominantly negative representations over a few years, reflecting broader societal attitudes.
Qualitative data analysis involves various procedures, with three major approaches identified by Miles and Huberman (1994): interpretative approaches, social anthropological approaches, and collaborative social research approaches.
Interpretative Approaches: This approach views human action and social activities as a collection of symbols with layered meanings. Researchers analyze transcribed interviews and observational data to uncover meanings and actions. The interpretation depends on the researcher's theoretical perspective. Phenomenological researchers, for example, focus on capturing the essence of an account, while others organize data to identify patterns in human activity and meaning. Interpretative approaches assume that all content, from interviews to photographs, is created for communication, allowing researchers to uncover surface and latent meanings and intents.
Social Anthropological Approaches: These approaches involve extensive field or case study activities, requiring researchers to spend considerable time with a study population. This immersion provides a unique perspective on collected material, including diaries, observations, interviews, photographs, and artifacts. The goal is to understand behavioral regularities, language use, rituals, and relationships in a specific setting. This approach emphasizes identifying patterns across multiple sources, rather than solely interpreting text from field notes.
Collaborative Social Research Approaches: In this mode, researchers work with subjects to effect change or action in a specific setting. The analysis of collected data is a collaborative process with the subjects, viewed as stakeholders. This approach involves reflexive consideration of data for feedback and understanding, and while it may use similar strategies to interpretative and social anthropology approaches, its goal is to generate a shared perspective from diverse sources.
Content Analysis and Theory: Hsieh and Shannon (2005) describe three qualitative content analysis approaches: conventional, directed, and summative. Conventional content analysis generates theories from coding categories derived directly from raw data. Directed content analysis uses codes from existing theories and raw data to explore meanings within a theoretical framework. Summative content analysis starts with counting existing words or phrases in the text and extends to explore latent meanings and themes
11.3: Examine how content analysis is used in research Content analysis is a research technique for making replicable and valid inferences from texts to their contexts. It applies to various qualitative data forms, like written documents, photographs, and videotapes, that can be "read." An example includes analyzing the Society of United Irishmen's membership oath through contemporary identity theories to reveal cultural nationalism. The method ensures systematic, nonarbitrary analysis, where explicit criteria for content coding and usage are established beforehand.
11.3.1: Quantitative vs Qualitative Content analysis can be both quantitative and qualitative. Quantitative content analysis might involve counts of textual elements, like the Bechdel Test for movies. In contrast, qualitative content analysis involves deeper interpretation of text context and meaning, such as the Trinity syndrome in film character analysis. Both approaches can provide different insights into the social communication being studied.
11.3.2: Manifest versus Latent Content Analysis This distinction is crucial in content analysis. Manifest content refers to elements that are physically present and countable, focusing on the surface structure of the message. Latent content analysis goes deeper, interpreting the underlying symbolism or subtext of the data. For instance, manifest analysis may count instances of violence in a novel, while latent analysis explores implied power dynamics and emotional impacts. This approach helps in understanding both the visible and deeper meanings within the data.
11.4: Analyze how the communication components are used in research Communication components in research include the message, sender, and audience. The message, conveying information (intended or not), is analyzed for themes, emphasis on topics, and space or time allocation. Sometimes, messages also provide insights about the sender. Strauss differentiates between in vivo codes (terms used by subjects) and sociological constructs (analytic categories like 'professional attitude'). Latent meanings in messages range from obvious to subtle, and their interpretation can vary among speakers and listeners.
11.4.1: Levels and Units of Analysis Content analysis of written documents requires deciding the level of sampling and units of analysis, which may range from words to entire books or series. For example, analyzing television programs might involve segments between commercials or entire episodes. In photographs, the analysis might focus on framing or the action depicted. Robert Fitts’ study of New York’s Five Points District is an example where the latent message about middle-class values was discerned from written descriptions.
11.4.2: Building Grounded Theory Content analysis categories can be inductively or deductively determined, or a combination of both. Inductive approach involves immersing in documents to identify meaningful themes, while deductive approach starts with a theoretical framework. Grounded theory, according to Glaser and Strauss, combines inductive and deductive reasoning, ensuring the theory fits the data and is understandable to laymen and sociologists alike.
11.4.3: What to Count Content elements include words, themes, characters, paragraphs, items, concepts, and semantics. These elements apply to both textual and visual content. Each element has its specific use, like words for frequency distribution or themes for sentences with a subject and predicate. Concepts group words into clusters representing ideas or variables.
11.4.4: Combinations of Elements Research often requires combining content analytic elements. Berg's study on Jewish affiliational categories used item and paragraph elements, analyzing respondents' definitions of each category from interviews.
11.4.5: Units and Categories Content analysis involves specifying content characteristics and applying rules for identifying and recording these. Categories vary based on research nature and data type, involving an interplay of theoretical concerns and empirical observations. Both inductive and deductive reasoning help develop these categories.
11.4.6: Classes and Categories Standard content analysis identifies classes and categories through common, special, and theoretical classes. Common classes are general cultural distinctions, special classes are specific to certain communities or professions, and theoretical classes emerge during data analysis, providing key linkages and patterns. Open coding is used to identify these classes and categories in data.
11.5 Content Analysis and Discourse Analysis
Content analysis and discourse analysis are closely linked in examining language patterns in communication and their social and cultural contexts. Discourse analysis studies everyday language, encompassing spoken, written, and non-verbal forms like sign language, focusing on the social construction of meanings. Content analysis builds on this by exploring the language used in communications, considering the cultural and social settings where these occur. It involves analyzing how discourse is structured and what it aims to convey in specific cultural contexts. This process often includes counting words, terms, and themes to understand how people construct their social worlds. This approach is applicable across various data sources, such as fieldwork, interviews, and focus groups, essential for revealing deeper insights into communication's social and cultural dimensions.
11.6 Open Coding
When conducting open coding in qualitative research, Strauss (1987) suggests four basic guidelines to help researchers navigate through common obstacles, such as interpreting the true meaning of sentences or the real motivation behind a subject’s statements:
Ask the Data Specific and Consistent Questions: Researchers should continually refer back to their original research objective, tailoring their open coding to align with the study's goals. For example, if researching moral advice in Harlequin romances, focus on identifying relevant principles or character morals, avoiding unrelated aspects unless they prove significant.
Analyze the Data Minutely: Open coding should start with a broad, inclusive approach, gradually becoming more refined. It’s important to code in detail initially, ensuring extensive coverage that later facilitates more systematic coding. The process can shift to more systematic coding once the data becomes saturated with repetitive codes.
Frequently Interrupt Coding to Write Theoretical Notes: Insights and ideas that arise during coding should be noted immediately. These notes can lead to new code categories or contribute to developing theories. For instance, observations about drug-crime relationships in interview transcripts might evolve into theories on their interplay.
Do Not Assume Analytic Relevance of Traditional Variables Until Proven by Data: Variables like age, sex, and social class should only be considered relevant if the data substantiates their importance. This approach ensures that any conclusions drawn are firmly grounded in the data itself.
Coding is key to organizing data and interpreting its meanings. There are various types of coding, such as descriptive, topic gathering, and analytic, each serving different analysis purposes. Regardless of the coding approach, it’s crucial to start analysis as data is collected, allowing patterns and categories to emerge naturally. This approach facilitates a more organic and accurate understanding of the research subject.
11.7: Examine the process of using coding frames in content analysis
Coding frames in content analysis are used to organize data and identify findings post-open coding. Initially, cases are sorted into specified classes, similar to axial coding as described by Strauss (1987). For instance, Berg (1983) sorted Jewish affiliational categories based on respondents' self-reports during telephone screening. This first level of sorting was followed by further subdivision based on criteria such as consistency with initial responses and the presence of qualifiers or detailed explanations. This process eventually led to a typological scheme with 16 categories.
11.7.1: A Few More Words on Analytic Induction
Analytic induction is used for theory development, contrasting with deductive reasoning, which tests theories. It involves seeking patterns that explain data, requiring a maximized search for negative or contradictory cases. Lindesmith and Merton highlight the importance of falsifiability in hypotheses. Glaser and Strauss recommend combining data analysis and theory integration, refining coding categories on the fly for an honest presentation of findings.
11.7.2: Interrogative Hypothesis Testing
Interrogative hypothesis testing involves actively searching for negative cases to test hypotheses. This process includes formulating a hypothesis, searching for negative cases, reformulating the hypothesis if necessary, and examining all cases for 'practical certainty'. Becker's 'null hypothesis trick' suggests initially assuming no patterns in data and disproving this assumption. However, researchers must guard against ignoring contradictory evidence or distorting the original hypothesis. Using safeguards like random selection of examples, multiple evidences for each assertion, independent review, and documenting inconsistencies can protect against these biases. This approach avoids 'exampling', which is finding examples for preconceived theories, and ensures a more systematic and reliable content analysis. Researchers should clearly explain their methods and adhere to them precisely to establish credibility in their findings.
Content Analysis Process Steps
Start with the Research Question: Determine what you are trying to explain. This forms the foundation of your content analysis.
Develop Analytic Categories: These categories are formed based on literature review, research questions, or as identified in interviews. They help in sorting the data into meaningful segments.
Read Through the Data: Engage with a substantial amount of the collected data, identifying relevant themes and categories that align with your research question. Note any recurring, unexpected patterns for future exploration.
Establish Objective Criteria for Coding: Define explicit rules or criteria for each category, both analytic and grounded. This could range from specific phrases to inferential levels. For instance, in analyzing memoirs of American jazz musicians, codes could relate to reasons for living in Harlem, such as the music scene or housing segregation.
Sort Data According to Criteria: Organize the data by applying the established criteria. This can be done manually with highlighters or sticky notes, or digitally using specialized content analysis software.
Count Data Chunks in Each Category: Take an initial look at the data by counting the instances in each category. This helps in identifying where to look for patterns and the strength of these patterns.
Examine Patterns Among Categories: Investigate how different ideas or codes are related. Check if certain ideas are consistently associated or if specific codes appear only in certain contexts. Look for patterns across various respondent groups or cases.
Explain Thematic Patterns: The final step involves explaining the identified patterns. Compare your findings with existing literature and theory to understand if they confirm or contradict previous research. Use this analysis to answer your research question, drawing upon the literature and your initial conceptualization of the study.
This step-by-step process ensures a thorough and systematic approach to content analysis, allowing for meaningful interpretation and understanding of the data in relation to the research question.
Computer Programs and Data Analysis
Various computer programs significantly aid qualitative research data analysis, offering diverse functionalities that enhance the research process:
Spreadsheet Programs: Tools like Excel or Lotus 1-2-3 are utilized for tasks in content analysis. They enable researchers to create fields for shorthand themes, classes, or categories and tally these categorical containers. Such spreadsheets can automatically calculate how frequently a theme appears in a category.
Word-Processing Programs: Some researchers use components of these programs to simulate functions of specialized qualitative data-analysis software, allowing for basic coding and organization of data.
Dedicated Qualitative Data-Analysis Software: These programs are designed for comprehensive content analysis, facilitating every stage of the process. They allow for storing materials, ideas, and theories, and offer features to enter and sort codes, add comments, run multiple analyses, and generate custom reports. This software is akin to quantitative analysis tools in functionality.
Facilitation of Team Projects: These software packages support collaborative efforts by creating a shared project space where all data files, codes, queries, and outputs are stored and managed, ensuring team members can work simultaneously without data overlap or loss.
Coding and Analysis Features: For instance, MAXQDA allows researchers to code text, insert paragraph numbers, and attach notes to specific sections. Users can search for data with specific codes and run summaries for individual cases.
Visual Displays: Qualitative software offers various interactive visual representations of data. Tools like ATLAS.ti can display network views showing links among different data segments, revealing connections and relationships.
Advanced Visualizations: Software like NVivo can generate word trees that relate code categories to their occurrences in the data, aiding in discovering and analyzing relationships within the data.
However, it's crucial to remember that the output from these software programs reflects the researcher's input in terms of code definitions, data associations, and query settings. The visualizations and analyses produced do not inherently reveal the "truth" of the data but rather the relationships and interpretations coded by the researchers. These tools are instrumental in navigating the vast expanse of qualitative research data, offering structured, systematic, and collaborative approaches to data analysis.
11.10: Advantages of Content Analysis
Unobtrusiveness: Content analysis is nonreactive and does not require interviewing people or conducting surveys. It relies on existing sources like newspapers, public addresses, archives, TV shows, and movies, allowing researchers to analyze data that wasn’t influenced by their research process.
Cost-Effectiveness: It is generally inexpensive and accessible. A single researcher can conduct a content analysis study, making it a more feasible option than, say, a national survey which requires significant resources.
11.11: Limitations of Content Analysis
Restricted to Recorded Messages: Content analysis is limited to analyzing pre-recorded messages, be they oral, written, graphic, or videotaped. This reliance on existing content means researchers can only examine records that others deemed worthy of preservation.
Invisible Filters in Data Pool: Before data collection, the pool of available data is already narrowed by unseen filters. For example, online comments on news websites may not represent the broader reader population and could skew toward certain sentiments due to the nature of internet discourse.
Ineffectiveness in Testing Causal Relationships: Content analysis often falls short in establishing causality between variables. While it can identify what is present in the data, it cannot explain why. Causal relationships may be inferred from patterns, but other methods are required to confirm these inferences.
Autoethnography: Learning about content analysis has been very enlightening. It is a lot! so kinda freaking out for my 15 page paper, wish I gave myself more time to complete it, but I really do find it very interesting, I especially love how much technology can help you to make things easier on yourself when it comes to transcribing.
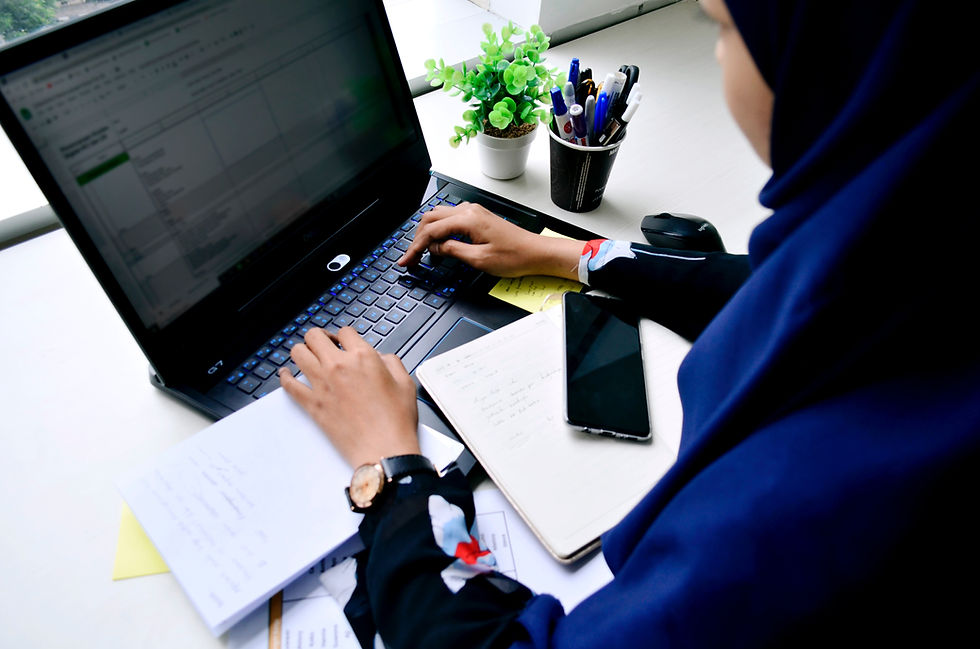
Works Cited
Lune, Howard. 2021. Qualitative Research Methods for the Social Sciences, 9th edition. Sage Publications.
Comments